Review written by Kimberly Sabsay (LSI, GS)
The transition to a highly digitized society is well underway. Hospital data and medical charts are no exception. According to the CDC, over 85% of healthcare organizations have adopted Electronic Health Record (EHR) systems as of 2017. EHRs, while increasingly complex, could very well hold the secret to advancing patient care and diagnostics. EHRs contain medical history, medications, and test results, much like a regular health record, while also providing real-time information and tools to automate treatment plans. Predictive healthcare analytics are at our fingertips, and a novel statistical framework designed by researchers at Princeton University unlocks the massive potential of personalized, predictive, and real-time medical monitoring systems.
Using EHR data to discover patterns for preventative care is not necessarily a foreign concept within the scientific community. Health care data is, by nature, sporadic and burdened with non-uniform gaps. In other words, there are random periods of time within a patient’s EHR with no data (i.e., when a patient is not receiving medical care) and other periods of time with ample data (i.e., when a patient has been admitted to a hospital due to an ailment). Additionally, due to both time and financial constraints, doctors are unable to order a plethora of clinical tests for every patient that comes to them. In practice, specific tests are performed to confirm a hypothesis deduced from the distinct symptoms a patient displays. This non-uniformity presents significant computational challenges for modeling the physiological progression of patient state. Recent efforts have focused on filling in the missing holes within medical record data in order to apply typical time series analyses which assume temporal regularity. Instead of “patching” up the sparse data, using Gaussian processes can organically capture irregular observations while also providing information regarding its own uncertainty in predictions. Essentially, Gaussian processes are distributions on smooth, nonlinear functions capturing the relationship between two or more variables, here, time vs clinical covariates. Practically, this statistical tool can model non-uniform events across time, like sporadic EHRs, as long as there is some smoothness to these observations. These processes are now being used to model medical time series data (i.e., patient condition vs. time) with success in predicting specific clinical covariates (lab test values and vital signs).
However, a majority of the previous work on this task has modeled each covariate independent of the rest. It should be no surprise that many covariates are highly correlated, like one’s heart rate and respiratory rate. The human body is a system, meaning studies that model each attribute of a patient’s physiological condition independent of any other are missing crucial information. Efforts are being made to push the boundaries of multivariate irregular modeling that will allow scientists to model non-uniform patient state based on a combination of clinical variables, rather than one vital sign or test result. Until now, only a few restrictive methods have been proposed. These methods are limited by their assumption of temporal regularity (consistent data collection across time), and thus require gaps in the data to be manually corrected or accounted for prior to modeling. In a recent publication, Sparse multi-output Gaussian processes for online medical time series prediction, Princeton COS Professor Barbara Engelhardt, along with graduate student Li-Feng Cheng, collaborators from the University of Pennsylvania hospital system, and other colleagues propose a unique Gaussian process regression framework, called MedGP, to model 24 clinical covariates and produce individualizable models for guiding future patients’ preventative care efforts.
The relative relationship between two variables is measured by the covariance--which can be thought of as similar to a correlation. This covariance function is called the kernel and determines the properties of a distribution, i.e., smoothness or periodicity. Choosing an appropriate kernel is essential in creating an accurate model. A combination of kernels is often used, and one version of this combination is referred to as the spectral mixture (SM) kernel. The SM kernel can capture irregular and semi-periodic medical time data. This framework allows researchers to model a patient’s irregular, dynamic time series data.
In order to construct a population-level empirical prior, or foundational baseline, large quantities of hospital record data were obtained from the Hospitals at the University of Pennsylvania (HUP) and the Public Multiparameter Intelligent Monitoring in Intensive Care (MIMIC-III). Three disease groups from HUP (sepsis, heart failure, and neoplasm patient groups) and one disease group from MIMIC-III (an additional heart failure patient group) were established on the basis of data availability.
By training Gaussian process kernels for each patient within the established disease groups, Cheng et al. estimated group-specific distributions of the hyperparameters that shape the trajectory of time series data. When a patient checks in to the hospital, their vital signs and lab test results are used to update the baseline kernel to create a personalized patient tool. The large amount of data used to generate the original hyperparameters makes updating these values for each new observation computationally demanding. If this system is to be used in a clinical setting, it should update each patient-specific kernel relatively quickly or else the predictive estimates may come too late to aid in a patient’s treatment. A momentum-based method is used to make this learning process practical.
MedGP’s performance for all four disease groups was evaluated alongside three previously designed algorithms for online monitoring of medical time series data: the independent GP model, the naïve one-lag model, and the multi-resolution Probability Subtyping Model (PSM). MedGP, which is an SM-LMC kernel with a sparse prior, provides substantial improvements in the estimation of the majority of the 24 covariates in all disease groups across all three competing methods (Figure 1). Additionally, MedGP with online sequential updating improves covariate predictions (compared to a model of MedGP without online updating) for at least 50% of the covariates for all four disease groups. The online updating essentially feeds the algorithm new information, sequentially, as it is obtained, preserving the order in which covariates are measured. This allows for the MedGP algorithm to continually “learn” as more information is acquired. The value of updating the model’s empirical prior knowledge with real-time patient-specific observations is evident from this discerned improvement.
As the authors note in their publication, this method can be further improved by incorporating hierarchical methods with features to represent differences between patients in even more detail. Regardless, this framework can be used to monitor the health of patients and simultaneously predict acute diseases, increasing the amount of information doctors have available for making critical decisions about patient care that could be the difference between life and death.
Princeton University researchers in Dr. Barbara Engelhardt’s lab developed this sophisticated and efficient method to model sparse, irregular, and time-dependent patient data. They successfully harnessed the power of Gaussian processes and substantially improved previous state-of-the-art techniques. MedGP provides robust estimates of future patient states by learning from the past and accounting for the present, thus spanning the entire dimension of time, for the betterment of human health.
Professor Barbara Engelhardt explains, “While there is a lot more work to do in real-time monitoring of hospital patient data, our hope is that MedGP might be the basis upon which decision-making support for clinician-in-the-loop clinical care tools are developed. Without these patient state models, it is hard to know how particular interventions will actually impact patient state.” She hopes that these tools will allow doctors to evaluate different treatment options for patients using a personalized model. So these tools will allow us to evaluate different options for a patient using a personalized model.”
This article was published in BMC Medical Informatics and Decision Making on July 8, 2020. Please follow this link to view the original version.
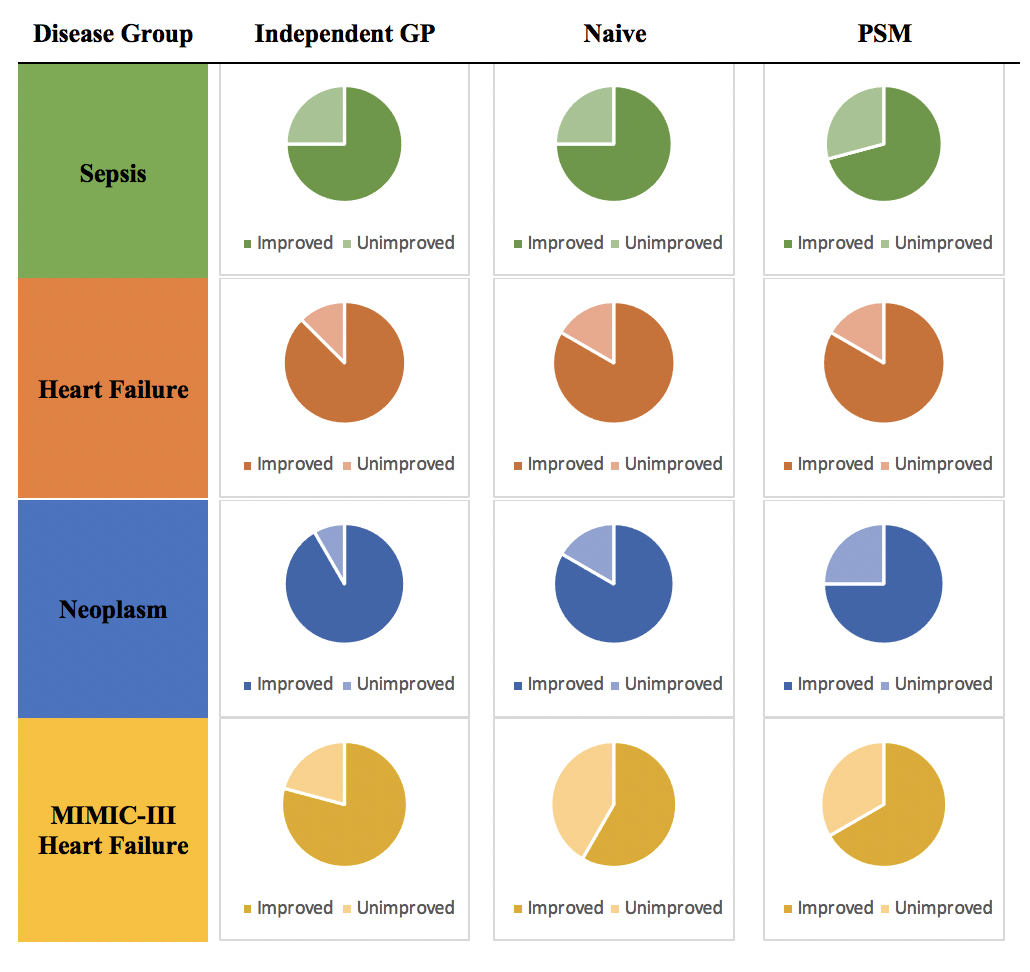
Original publication:
Cheng, L., Dumitrascu, B., Darnell, G. et al. Sparse multi-output Gaussian processes for online medical time series prediction. BMC Med Inform Decis Mak 20, 152 (2020). https://doi.org/10.1186/s12911-020-1069-4