Review by Tamjeed Azad (COS, G1)
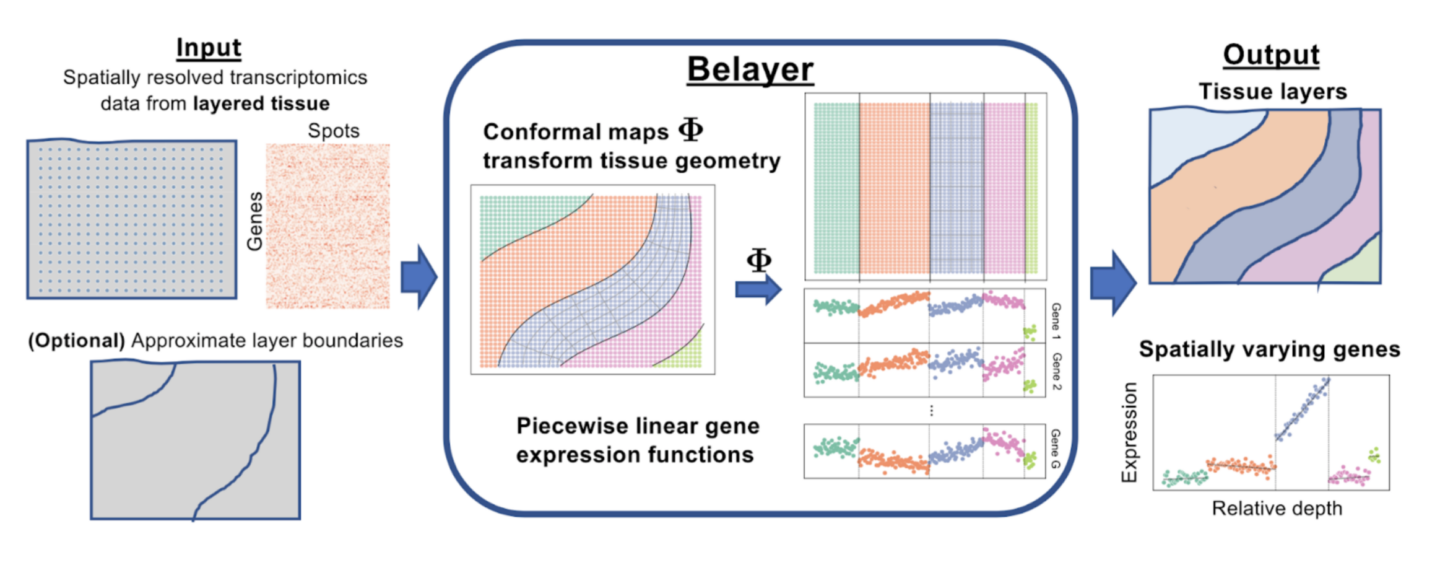
All the marvelous biology we see, from the smallest bugs to the largest trees, is driven by behavior, function, and characteristics of individual cells. The activity of these cells is driven by molecules called proteins, and different cells derive their different characteristics by which specific proteins they use to carry out necessary biological processes. The proteins that are built within cells are determined by which genes of an organism’s DNA are decoded and transcribed into RNA, the blueprint for protein construction. Modern techniques in genetics measure which genes in a tissue are transcribed, in an effort to infer the drivers of such tissues’ biological activity and thus elucidate which functional characteristics are important in a given tissue. Spatially resolved transcriptomics, a subset of these methods, lets biologists and practitioners view which genes are transcribed and to what level (called gene expression) but in a spatial context, making it clear what location within a tissue slice each gene is expressed.
Modern spatial transcriptomics assays generally suffer from technical noise and under-detection of the actual number of genes transcribed, despite returning information for a large number of genes and spatial locations (which can reach cellular resolution); this complexity demands the need for explicit computational modeling of spatial transcriptomics data, in order to distinguish between technical and true biological variation in a unified manner. Methods exist to explain through prediction, or model, the variability of gene expression within these datasets, and current models for spatial data choose one of two options, either assuming that gene expression varies in a discrete fashion, where cells form individual, similar groups of similar expression, or that expression of genes varies continuously, where gene expression changes in a gradient-like fashion across spatial locations.
A team of researchers at Princeton, co-led by Dr. Cong Ma and Uthsav Chitra in the lab of Dr. Ben Raphael, has developed a new method, Belayer, which aims to model spatial transcriptomics data from a tissue slice that contains multiple physical biological layers of tissue types. To accomplish this, the model allows for explicit modeling of both discrete and continuous variations in gene expression. Ma et al. created Belayer with three defining characteristics. Firstly, expression of each gene is modeled as a piecewise linear function of the relative depth within each tissue layer. Here, the relative depth is a quantity describing the position from one layered end of the tissue to the other end, and the piecewise linear function models this expression using different linear functions over different intervals of these positions. Secondly, a tool from complex analysis called a conformal map is used to transform the geometry of the naturally biologically curved tissue layers to vertical layers, in order to maintain a consistent and linear concept of relative depth in a tissue. And lastly, a dynamic programming algorithm is used to learn what these layers and piecewise linear gene expression functions are.
The performance of Belayer is compared against that of other analytical methods used in the field of bioinformatics for spatial transcriptomics data, specifically SpaGCN, stLearn, and BayesSpace [1,2,3]. The researchers used computer generated simulations to compare the performance of Belayer against BayesSpace and SpaGCN, as well as SCANPY, without spatial information [4]. Two simulations of layered, spatially resolved tissue data were used, one directly generated from Belayer’s piecewise linear model, and another from the package Splatter [5]. Under both simulations, Belayer outperforms the other methods by identifying the correct layers of tissue more accurately than them.
While the Belayer model performed well against other computer modeling approaches with simulations, Ma et al also wanted to test its accuracy on datasets generated from real tissue. To do this, they evaluated Belayer’s performance on three non-simulated datasets: one collected from the human dorsolateral prefrontal cortex, the area of our brains contributing to our ability to perform executive tasks, another from the mouse skin during wound healing, and a third from the mouse somatosensory cortex, the brain region associated with the perception of the senses. In all three of the real datasets, Belayer more correctly identifies different layers of cell types than the other methods.
The conclusions of this study are exciting, as it demonstrates the power of using both continuous and discrete modeling paradigms in modeling a variety of naturally layered biological tissue. Also, multiple extensions and improvements to Belayer in the future could occur, such as extending the idea of tissue layers to more complex geometries, such as concentric or striated layers of muscle tissue. This would allow Belayer to be applied more broadly to additional spatial datasets and biological settings. Advances such as Belayer and its subsequent extensions are evidently promising for enabling a more precise and accurate interpretation of tissues, organs, and general biological systems, making it amazing to consider how far methods such as these will propel our understanding of biology forward.
The original article discussed here and the accompanying figure was published in Cell Systems on October 19, 2022. Please follow this link to view the full version.
References:
[1] Hu, J., Li, X., Coleman, K., Schroeder, A., Ma, N., Irwin, D.J., Lee, E.B., Shinohara, R.T., and Li, M. (2021). SpaGCN: integrating gene expression, spatial location and histology to identify spatial domains and spatially variable genes by graph convolutional network. Nat. Methods 18, 1342–1351.
[2] Zhao, E., Stone, M.R., Ren, X., Guenthoer, J., Smythe, K.S., Pulliam, T., Williams, S.R., Uytingco, C.R., Taylor, S.E.B., Nghiem, P., et al. (2021). Spatial transcriptomics at subspot resolution with BayesSpace. Nat. Biotechnol. 39, 1375–1384.
[3] Pham, D., Tan, X., Xu, J., Grice, L.F., Lam, P.Y., Raghubar, A., Vukovic, J., Ruitenberg, M.J., and Nguyen, Q. (2020). stLearn: integrating spatial location, tissue morphology and gene expression to find cell types, cell-cell interactions and spatial trajectories within undissociated tissues. bioRxiv.
[4] Wolf, F.A., Angerer, P., and Theis, F.J. (2018). SCANPY: large-scale single-cell gene expression data analysis. Genome Biol. 19, 15.
[5] Zappia, L., Phipson, B., and Oshlack, A. (2017). Splatter: simulation of single- cell RNA sequencing data. Genome Biol. 18, 174.